Ontology-based Categorization of Clinical Studies
a method for automated ontology-based categorization of clinical studies by their conditions
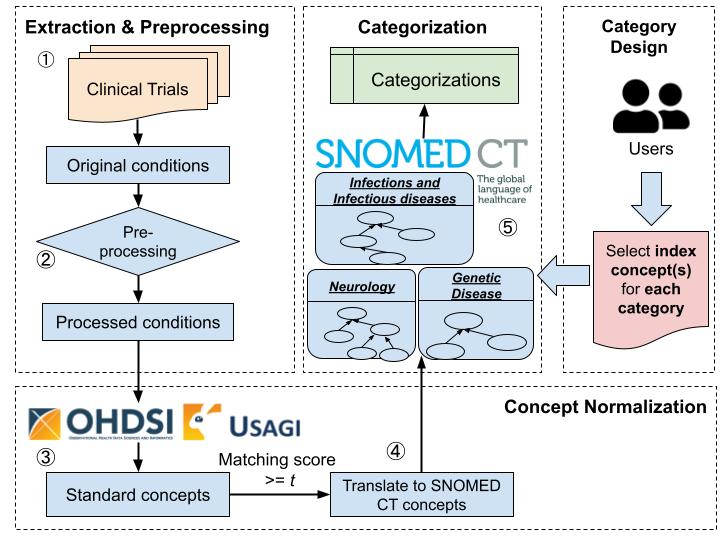
- (1) an information extraction module for extracting concepts from the free-text Condition field and performing necessary preprocessing;
- (2) a concept normalization module for automatic generation of standardized concepts using Usagi and OMOP CDM standard vocabularies;
- (3) a categorization module for automatically classifying a study to categories.
The source code of this project is available at https://github.com/dr-haoliu/ontology-based-clinical-study-categorization
-
Objective The free-text Condition data field in the ClinicalTrials.gov is not amenable to computational processes for retrieving, aggregating and visualizing clinical studies by condition categories. This paper contributes a method for automated ontology-based categorization of clinical studies by their conditions.
-
Materials and Methods Our method first maps text entries in ClinicalTrials.gov’s Condition field to standard condition concepts in the OMOP Common Data Model by using SNOMED CT as a reference ontology and using Usagi for concept normalization, followed by hierarchical traversal of the SNOMED ontology for concept expansion, ontology-driven condition categorization, and visualization. We compared the accuracy of this method to that of the MeSH-based method.
-
Results We reviewed the 4,506 studies on Vivli.org categorized by our method. Condition terms of 4,501 (99.89%) studies were successfully mapped to SNOMED CT concepts, and with a minimum concept mapping score threshold, 4,428 (98.27%) studies were categorized into 31 predefined categories. When validating with manual categorization results on a random sample of 300 studies, our method achieved an estimated categorization accuracy of 95.7%, while the MeSH-based method had an accuracy of 85.0%.
-
Conclusion We showed that categorizing clinical studies using their Condition terms with referencing to SNOMED CT achieved a better accuracy and coverage than using MeSH terms. The proposed ontology-driven condition categorization was useful to create accurate clinical study categorization that enables clinical researchers to aggregate evidence from a large number of clinical studies.
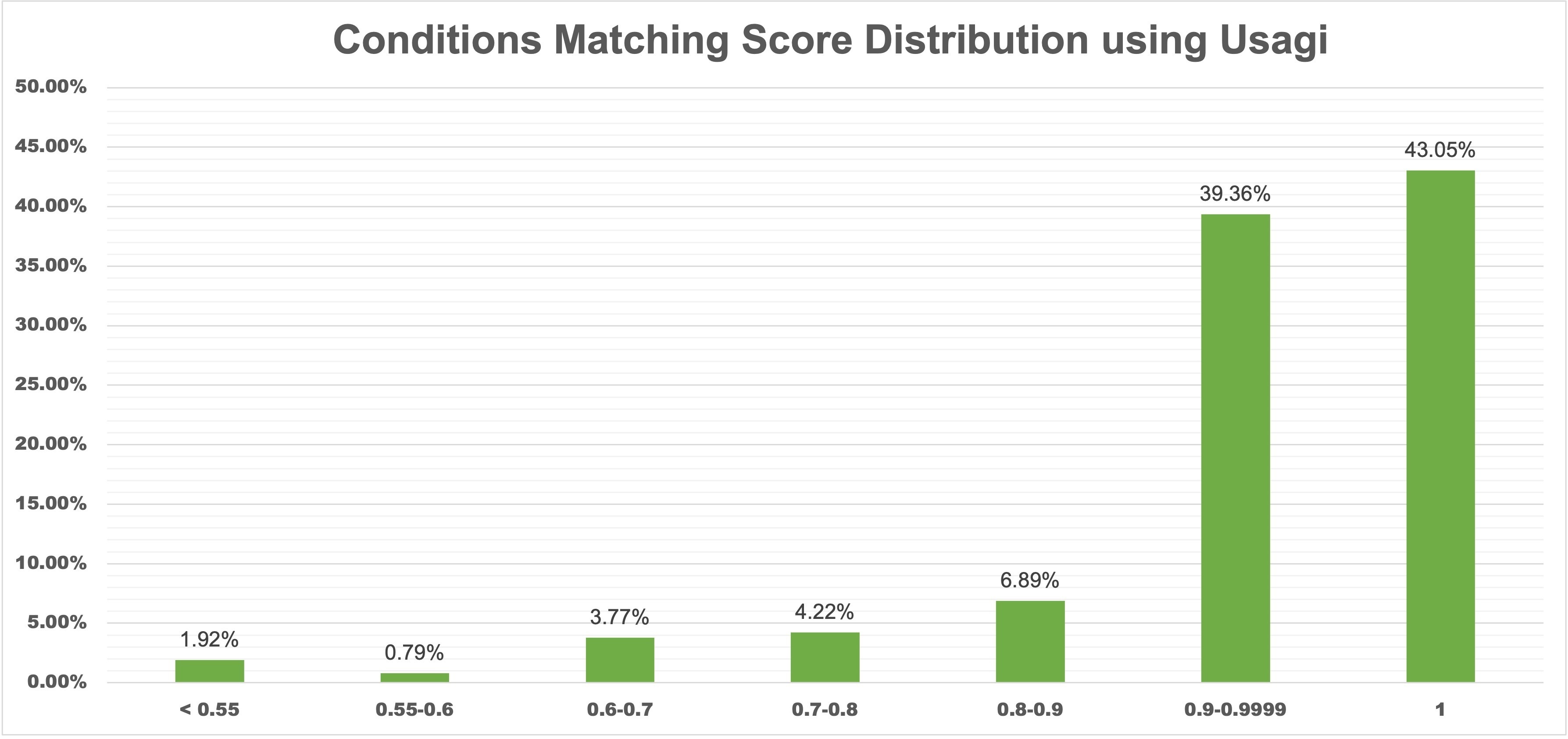
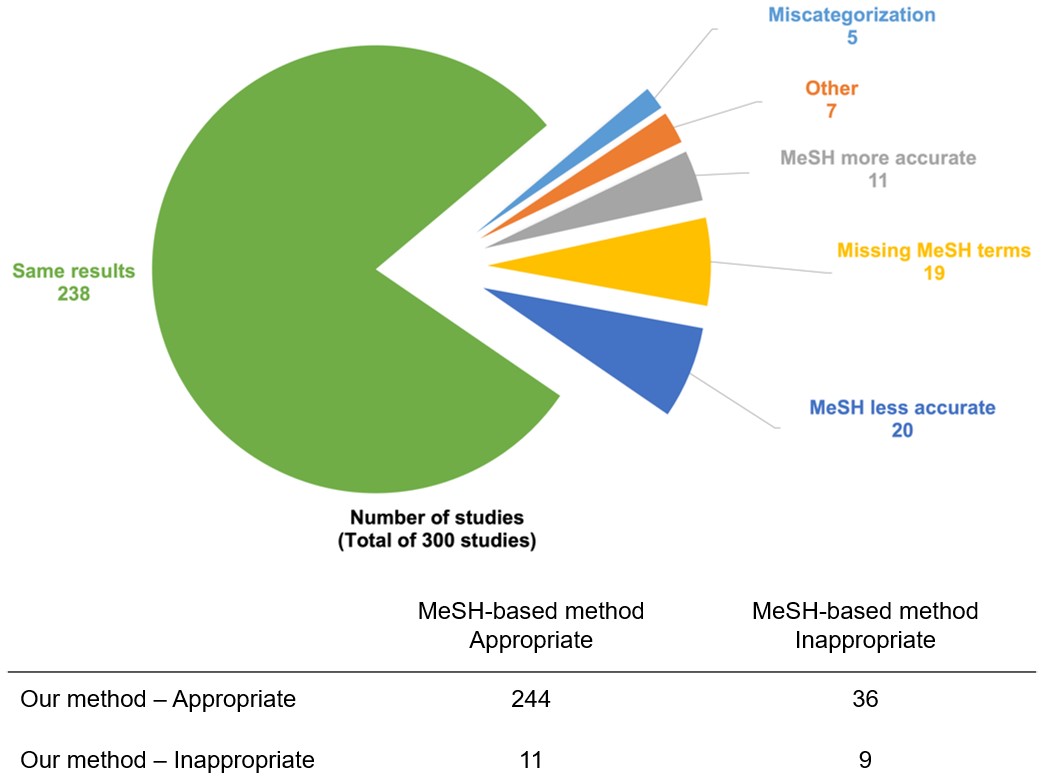